Markdown is thought as a “lightweight” markup language, hence the name
markdown
. That’s why formatting options are scarce. However, there are some
extensions, for instance brought by RMarkdown.
One point of particular interest is the sizing of figures. Let’s look at some ways how to size a figure with RMarkdown.
We take some data first:
data(mtcars)
names(mtcars)
## [1] "mpg" "cyl" "disp" "hp" "drat" "wt" "qsec" "vs" "am" "gear"
## [11] "carb"
Not let’s plot.
Define size in YAML header
We can define the size of figures globally in the YAML part, like this for example.
---
title: "My Document"
output: html_document:
fig_width: 6
fig_height: 4
---
Defining figures size for R plots
Define figure size as global chunk option
As a first R-chunk in your RMD document, define the general chunk settings like this:
knitr::opts_chunk$set(fig.width=12, fig.height=8)
Chunk options
We can set the chunk options for each chunk too. With figh.height
and
fig.width
we can define the size. Note that the numbers default to inches as
unit: {r fig1, fig.height = 3, fig.width = 5}
.
plot(pressure)
For a plot of different size, change simple the numbers: {r fig2, fig.height = 3, fig.width = 3, fig.align = "center"}
.
plot(pressure)
Alternatively, you may change the aspect ratio of the image: {r fig3, fig.width = 5, fig.asp = .62}
.
plot(pressure)
Note that the aspect ratio is based on the fig.width
specified by you. See
here.
Different options for different output formats
The options for figure sizing also depend on the output format (HTML vs. Latex, we do not mention Word here). For instance, in Latex percentage is allowed, as is specified on the options
page: {r fig4, out.width = '40%'}
.
plot(pressure)
But note that it appears to work in HTML too.
Differnce between figure size and output size
We are allowed to specify the
figure size, and secondly the size of the figure as to appear in the output. For
example, if you set the size of a ggplot
figure to large, then fonts etc. will
appear tiny. Better do not scale up fig.height
, but set out.width
accordingly, eg., like this out.width = "70%"
.
Using Pandoc’s Markdown for figure sizing
Alternatively, instead of using R
for plotting, you can just load an image. Of course, it is possible to just use
markdown for that: 
.
Change the figure size like this: { width=50% }
. Note that no
space are allowed around the =
(equal sign), and the curly brace {
needs to
come right after the )
brace; no space allowed.
Similarly, with path to local folder:

{
width=20% }
Centering is not really part of markdown. But there are some workarounds. See:
I used this code:
<center>
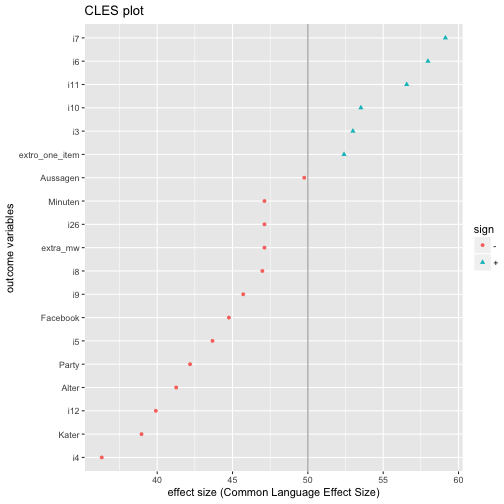{
width=20% }
</center>
Using the knitr
function include_graphics
We can use the knitr function include_graphics
which is convenient, as it takes care for the different output formats and provides some more features (see
here the help
file).
Note that online sources are allowed. Don`t forget to load knitr previously.
If all fails
Just resize the image with your favorite photo/image manager such as Gimp, Photoshop, Preview App etc.
Further reading Finde good advice on Yihui’s option page
here. The Book “R for Data Science” by Hadley Wickham and Garrett Grolemund (read here) is a great resource too. Read chapter 28 on diagrams here. Pandoc’s user guide has some helpful comments on figures sizing with Pandoc’s markdown .