Plotting (and more generally, analyzing) survey results is a frequent endeavor. Let’s not think about arguments whether and when surveys are useful (for some recent criticism see the Briggs’ book.
Typically, respondents circle some option ranging from “don’t agree at all” to “completely agree” for each question (or “item”). Typically, four to six boxes are given where one is expected to tick one.
In this tutorial, I will discuss some barplot type visualizations. Sure, much more can be done, but we will stick to this one plot for this post.
Some needed packages.
library(tidyverse)
library(forcats)
Prepare data
So, first, let’s load some data. That’s a data set of a survey on extraversion. Folks were asked a bunch of questions tapping at their “psychometric extraversion”, and some related behavior, that is, behavior supposed to be related such as “number of Facebook friends”, “how often at a party” etc. Note that college students form the sample.
Data are available only (free as in free and free as in beer).
data <- read.csv("https://sebastiansauer.github.io/data/extra_raw_WS16.csv")
data_raw <- data # backup just in case
OK, we got ‘em; a dataset of dimension 501, 24. Let’s glimpse at the data:
## Observations: 501
## Variables: 24
## $ Zeitstempel <fctr> ...
## $ Bitte.geben.Sie.Ihren.dreistellen.anonymen.Code.ein..1...Anfangsbuchstabe.des.Vornamens.Ihres.Vaters..2...Anfangsbuchstabe.des.Mädchennamens.Ihrer.Mutter..3..Anfangsbuchstabe.Ihres.Geburstsorts. <fctr> ...
## $ Ich.bin.gerne.mit.anderen.Menschen.zusammen. <int> ...
## $ Ich.bin.ein.Einzelgänger..... <int> ...
## $ Ich.bin.in.vielen.Vereinen.aktiv. <int> ...
## $ Ich.bin.ein.gesprächiger.und.kommunikativer.Mensch. <int> ...
## $ Ich.bin.sehr.kontaktfreudig. <int> ...
## $ Im.Grunde.bin.ich.oft.lieber.für.mich.allein..... <int> ...
## $ Ich.kann.schnell.gute.Stimmung.verbreiten. <int> ...
## $ Ich.gehe.gerne.auf.Partys. <int> ...
## $ Ich.bin.unternehmungslustig. <int> ...
## $ Ich.stehe.gerne.im.Mittelpunkt. <int> ...
## $ Wie.viele.Facebook.Freunde..Kontakte..haben.Sie..wenn.Sie.nicht.bei.Facebook.sind..bitte.LEER.lassen.. <int> ...
## $ Wie.viele..Kater...überreichlicher.Alkoholkonsum..hatten.Sie.in.den.letzten12.Monaten. <fctr> ...
## $ Wie.alt.sind.Sie. <int> ...
## $ Bitte.geben.Sie.Ihr.Geschlecht.an. <fctr> ...
## $ Ich.würde.sagen..ich.bin.extrovertiert. <int> ...
## $ Sie.gehen.alleine.auf.eine.Party..Nach.wie.viel.Minuten.sind.Sie.im.Gespräch. <fctr> ...
## $ Es.wird.ein.Mitarbeiter..m.w..für.eine.Präsentation..Messe..gesucht..Melden.Sie.sich.freiwillig. <fctr> ...
## $ Wie.häufig.waren.Sie.in.den.letzten.12.Monaten.auf.einer.Party. <fctr> ...
## $ Wie.oft.haben.Sie.Kundenkontakt. <fctr> ...
## $ Passt.die.folgende.Beschreibung.auf.Sie...........Eine.extravertierte.Person.ist.jemand..der.seine.Energie.eher.nach.außen.richtet.und.weniger.in.die.innere.Welt.der.Gedanken..Ideen.oder.Vorstellungen..Daher.neigen.extravertierte.Menschen.dazu..in.neuen.Situationen.ohne.zu.zögern.sich.in.die.neue.Situationen.zu.begeben..Zum.Beispiel.würde.eine.extravertierte.Person..die.zum.ersten.Mal.einen.Yogakurs.besucht..sich.nicht.scheuen..direkt.bei.den.Übungen.mitzumachen..Oder.wenn.eine.extravertierte.Person.eine.Kneipe.zum.ersten.besucht..würde.sie.sich.nicht.unbehaglich.fühlen..Man.kann.daher.sagen..dass.extravertierte.Personen.als.aktiv.wahrgenommen.werden.und.sich.zu.Unternehmungen.hingezogen.fühlen..bei.denen.sie.mit.anderen.Personen.in.Kontakt.kommen. <fctr> ...
## $ X <lgl> ...
## $ Wie.sehr.treffen.die.folgenden.Aussagen.auf.Sie.zu..Bitte.denken.Sie.dabei.nicht.an.spezifische.Situationen..sondern.ganz.allgemein..wie.sehr.die.Aussagen.Sie.selbst.in.den.meisten.Bereichen.und.Situationen.in.Ihrem.Leben.beschreiben.Allgemein.wirke.ich.tendenziell.eher.wie.eine.Person..die...... <int> ...
Renaming
Looks like a jungle. Now what? Let’s start with renaming the columns (variables).
extra_colnames <- names(data) # save names in this vector
names(data)[3:12] <- paste("i", formatC(1:10, width = 2, flag = "0"), sep = "")
Now columns 3 to 12 are now named “i1”, “i2”, etc. These columns reflect the items of a extraversion questionnaire.
names(data)[1] <- "timestamp"
names(data)[2] <- "code"
names(data)[13] <- "n_facebook_friends"
names(data)[14] <- "n_hangover"
names(data)[15] <- "age"
names(data)[16] <- "sex"
names(data)[17] <- "extra_single_item"
names(data)[18] <- "time_conversation"
names(data)[19] <- "presentation"
names(data)[20] <- "n_party"
names(data)[21] <- "clients"
names(data)[22] <- "extra_vignette"
names(data)[24] <- "extra_description"
data$X <- NULL
Recoding
Importantly, two items are negatively coded; we need to recode them (ie., “yes” gets “no”, and vice versa).
rename(data,
i04r = i04,
i08r = i08) -> data
data %>%
mutate(i04r = recode(i04r, `1` = 4L, `2` = 3L, `3` = 2L, `4` = 1L),
i08r = recode(i08r, `1` = 4L, `2` = 3L, `3` = 2L, `4` = 1L)) -> data
I suspect there are rows with no values at, complete blank. Let’s compute the proportion of NAs per row.
## [1] 1 1 1 1 1 1 1 1 1 2 1 1 1 1 1 1 1 2 1 1 1 1 1
## [24] 2 1 2 2 1 1 1 1 1 1 1 1 1 1 2 1 2 1 1 1 1 1 1
## [47] 1 1 2 1 1 3 1 1 1 1 1 1 1 1 1 1 1 1 1 1 1 1 1
## [70] 1 1 1 1 1 1 1 2 1 1 1 1 1 1 1 1 1 1 1 1 2 2 1
## [93] 1 2 1 1 1 2 2 1 1 2 1 1 3 1 1 1 1 2 1 2 1 1 1
## [116] 0 0 0 0 0 0 0 1 0 0 0 0 0 0 0 0 1 0 0 1 0 1 0
## [139] 1 0 0 1 0 0 0 0 0 0 1 0 0 0 0 0 0 0 0 1 0 0 2
## [162] 0 0 0 0 0 1 1 0 0 0 0 0 0 1 0 1 0 1 0 0 1 1 1
## [185] 1 0 3 0 2 0 0 0 0 0 0 1 1 0 0 0 0 1 1 0 0 0 0
## [208] 0 0 0 0 0 0 0 0 0 0 0 0 1 0 0 0 0 1 0 0 0 1 1
## [231] 1 1 0 1 1 0 1 1 0 0 0 1 0 0 0 0 0 0 0 0 0 0 0
## [254] 0 0 0 0 0 0 0 0 0 0 1 0 0 0 0 1 0 0 0 0 0 0 0
## [277] 0 1 0 1 0 0 0 1 0 0 0 0 0 0 0 0 0 0 0 1 0 0 0
## [300] 0 0 0 0 0 0 1 0 0 1 0 0 0 0 0 0 2 0 0 0 0 0 0
## [323] 1 0 0 1 0 0 0 0 0 0 0 1 0 0 0 0 0 0 1 1 0 0 0
## [346] 0 0 0 1 0 0 2 0 0 0 0 1 1 0 0 0 0 0 0 0 0 1 0
## [369] 0 0 1 0 0 0 0 0 0 0 1 1 0 0 0 0 1 0 0 0 0 0 1
## [392] 0 1 0 1 0 0 0 0 0 0 0 0 0 0 0 0 0 0 1 0 0 0 0
## [415] 0 0 0 0 0 0 0 0 0 0 1 1 0 0 0 0 0 0 0 1 0 0 0
## [438] 0 0 0 1 1 0 1 0 0 0 0 0 0 0 0 14 1 1 0 1 0 1 0
## [461] 0 0 0 0 0 11 0 0 0 1 0 0 0 1 1 0 1 1 0 0 0 0 0
## [484] 0 0 0 0 0 0 0 0 0 0 0 0 1 1 0 0 1 0
table(data$extra_description)
##
## 1 2 3 4 5 6 7 8 10
## 31 118 98 68 44 12 5 1 1
data %>%
mutate(prop_na_per_row = rowSums(is.na(data))/ncol(data)) -> data
count(data, prop_na_per_row)
## # A tibble: 6 × 2
## prop_na_per_row n
## <dbl> <int>
## 1 0.00000000 304
## 2 0.04347826 171
## 3 0.08695652 21
## 4 0.13043478 3
## 5 0.47826087 1
## 6 0.60869565 1
Rowwise means for survey etc.
Let’s compute the mean and the median extraversion.
data %>%
mutate(extra_mean = rowMeans(.[3:12], na.rm = TRUE),
extra_median = apply(.[3:12], 1, median, na.rm = TRUE)) -> data
Cleaning data
The number of hangovers should be numeric, but it isn’t. Let’s see.
count(data, n_hangover) %>% kable
n_hangover |
n |
|
13 |
0 |
98 |
1 |
59 |
10 |
38 |
100 |
1 |
106 |
4 |
12 |
18 |
15 |
10 |
153 |
1 |
18 |
1 |
2 |
63 |
20 |
14 |
200 |
1 |
24 |
8 |
25 |
5 |
28 |
1 |
3 |
42 |
30 |
11 |
35 |
1 |
4 |
25 |
40 |
9 |
48 |
2 |
5 |
38 |
50 |
6 |
6 |
15 |
7 |
2 |
70 |
2 |
8 |
7 |
80 |
1 |
9 |
2 |
98 |
1 |
ca. 18-23 |
1 |
Keinen |
1 |
OK, let’s parse the numbers only; a typical problem in surveys is that respondent do not give numbers where you would like them to give numbers (some survey tools allow you to control what the respondent may put in the field).
data$n_hangover <- parse_number(data$n_hangover)
## Warning: 2 parsing failures.
## row col expected actual
## 132 -- a number
## 425 -- a number .
data$time_conversation <- parse_number(data$time_conversation)
## Warning: 1 parsing failure.
## row col expected actual
## 153 -- a number
data$n_party <- parse_number(data$n_party)
## Warning: 1 parsing failure.
## row col expected actual
## 270 -- a number
data$n_clients <- parse_number(data$clients)
## Warning: 234 parsing failures.
## row col expected actual
## 34 -- a number
## 37 -- a number
## 38 -- a number
## 39 -- a number
## 42 -- a number
## ... ... ........ ......
## See problems(...) for more details.
Checking NA’s for items
data %>%
select(i01:i10) %>%
gather %>%
filter(is.na(value)) %>%
count
## # A tibble: 1 × 1
## n
## <int>
## 1 24
Hm, let’s see in more detail.
data %>%
select(i01:i10) %>%
filter(!complete.cases(.))
## i01 i02 i03 i04r i05 i06 i07 i08r i09 i10
## 1 4 1 3 NA 4 2 3 2 3 2
## 2 4 2 3 2 2 1 NA 1 4 3
## 3 3 2 1 3 3 2 2 4 3 NA
## 4 3 2 1 NA 3 3 3 3 3 1
## 5 4 4 1 1 NA 4 4 2 3 2
## 6 3 NA 2 2 2 1 2 3 4 2
## 7 NA NA NA NA NA NA NA NA NA NA
## 8 NA NA 2 3 NA NA NA NA NA NA
Hm, not so many cases have NAs. Let’s just exclude them, that’s the easiest, and we will not lose much (many cases, that is).
data %>%
select(i01:i10) %>%
filter(complete.cases(.)) %>% nrow
data %>%
select(i01:i10) %>%
na.omit -> data_items
Plotting item distribution
Typical stacked bar plot
The most obvious thing is to plot the distribution of the items (here: 10) of the survey. So let’s do that with ggplot
.
On the x-axis we would like to have each item (i1, i2, …), and on the y-axis the frequency of each answer, in some stapled bar fashion. That means, on the x-axis is one variable only. That’s why we need to “melt” the items to one “long” variable.
data_items %>%
gather(key = items, value = answer) %>%
mutate(answer = factor(answer)) %>%
ggplot(aes(x = items)) +
geom_bar(aes(fill = answer), position = "fill") -> p1
p1
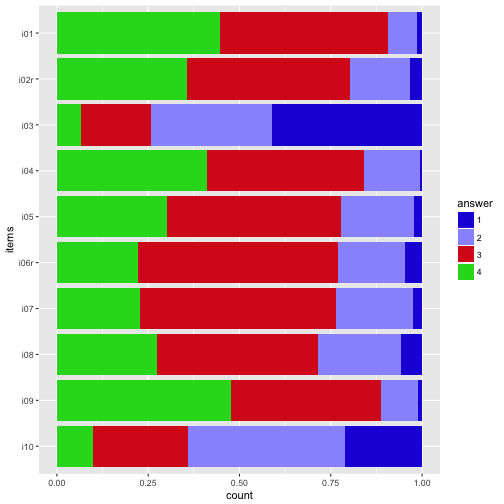
Maybe nicer to turn it 90 degrees:
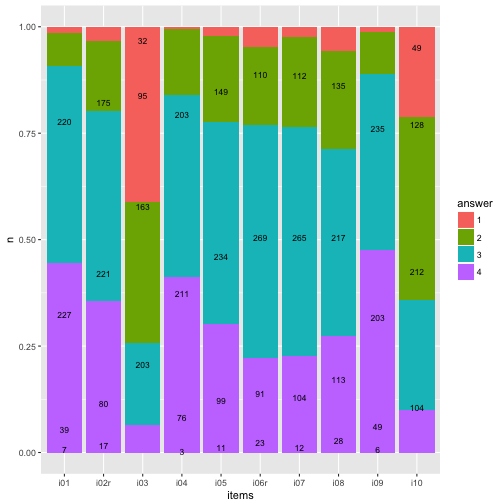
And reverse the order of the items, so that i01
is at the top.
data_items %>%
gather(key = items, value = answer) %>%
mutate(answer = factor(answer),
items = factor(items)) -> data2
ggplot(data2, aes(x = items)) +
geom_bar(aes(fill = answer), position = "fill") +
coord_flip() +
scale_x_discrete(limits = rev(levels(data2$items))) -> p2
p2
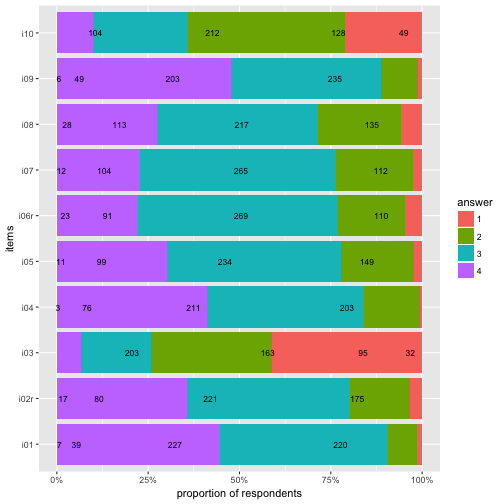
Colors
The beauty of the colors lie in the eye of the beholder… Let’s try a different palette.
Sequential color palettes.
p2 + scale_fill_brewer(palette = "Blues")
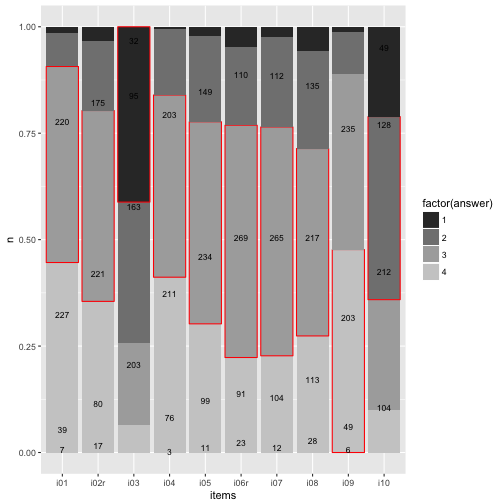
p2 + scale_fill_brewer(palette = "BuGn")
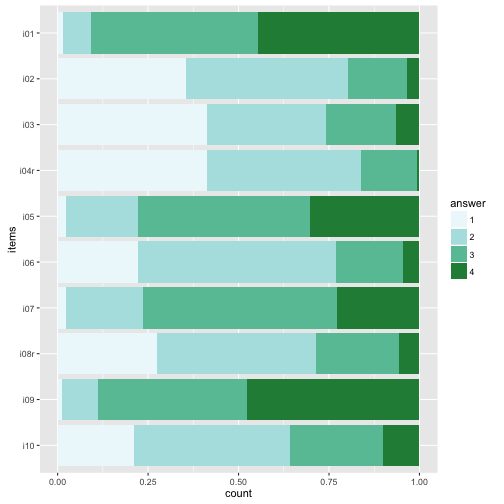
p2 + scale_fill_brewer(palette = 12)
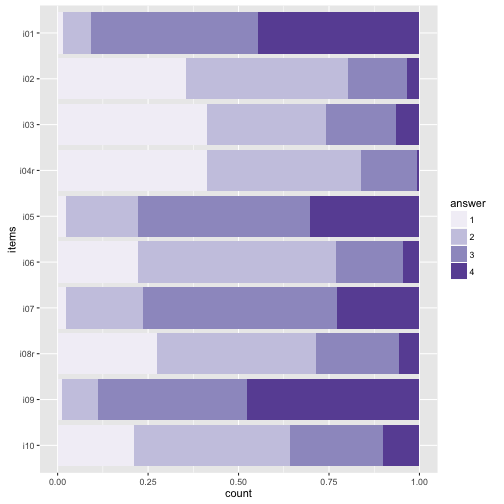
Diverging palettes.
p2 + scale_fill_brewer(palette = "RdYlGn")
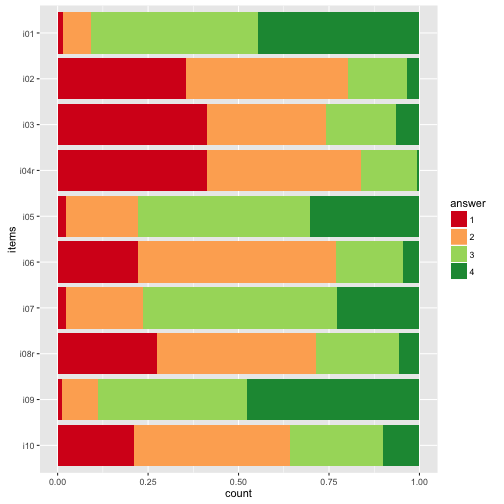
p2 + scale_fill_brewer(palette = "RdYlBu")
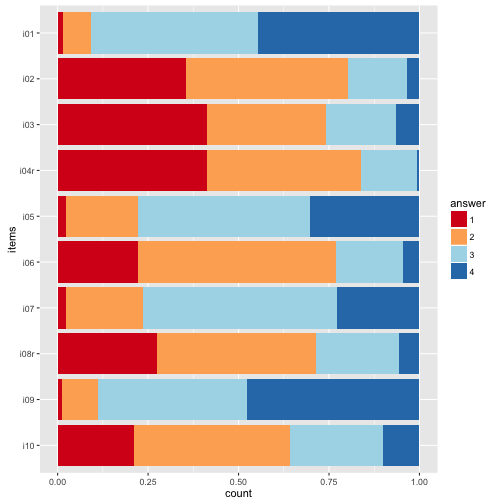
The divering palettes are useful because we have two “poles” - “do not agree” on one side, and “do agree” on the other side.
See here for an overview on Brewer palettes.
Or maybe just grey tones.
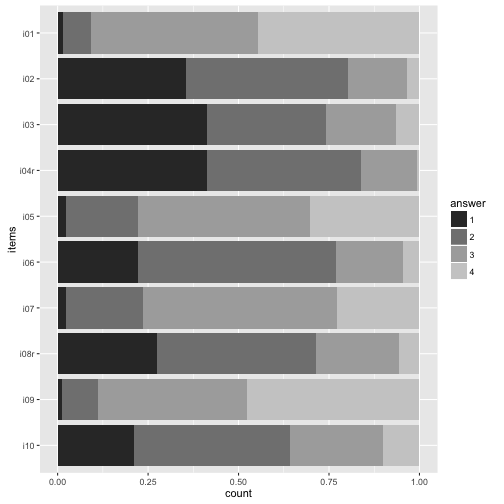
My own favorite-cherished color.
colours <- c("#2121D9", "#9999FF", "#D92121", "#21D921", "#FFFF4D", "#FF9326")
p2 + scale_fill_manual(values=colours)
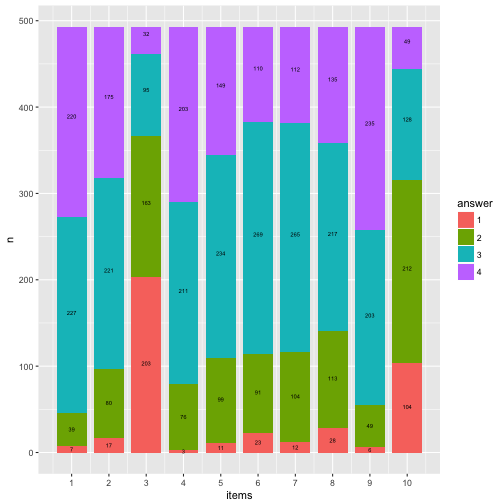
Numbers (count) on bars
It might be helpful to print the exact numbers on the bars.
data2 %>%
dplyr::count(items, answer) %>%
mutate(y_pos = cumsum(n)/nrow(data_items) - (0.5 * n/nrow(data_items)),
y_cumsum = cumsum(n)) %>%
mutate(items_num = parse_number(items)) -> data3
ggplot(data3, aes(x = items, y = n)) +
geom_bar(aes(fill = answer), position = "fill", stat = "identity") +
geom_text(aes(y = y_pos, label = n), size = 3) -> p3
p3
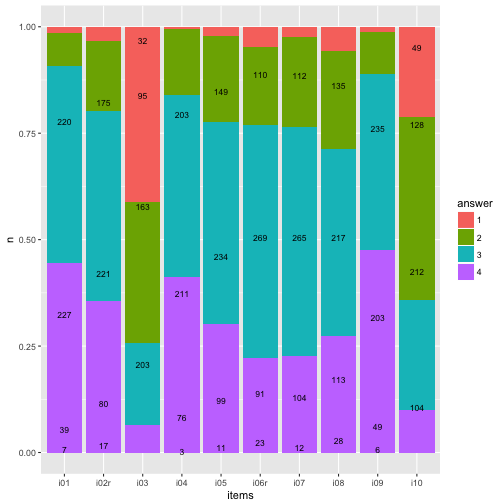
Flip coordinate system, and with “%” labels.
p3 + coord_flip() +
scale_y_continuous(labels = scales::percent) +
ylab("proportion of respondents") -> p4
p4
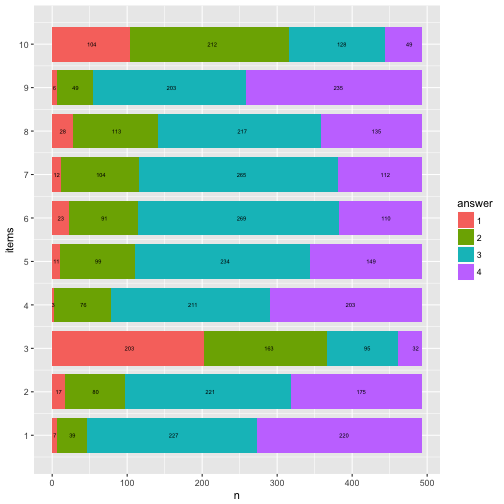
Highlight main category
data3 %>%
mutate(answer = as.numeric(answer)) %>%
group_by(items) %>%
mutate(max_n = max(n)) %>%
mutate(max_cat = factor(which(n == max_n))) %>%
mutate(fit_cat = (answer == max_cat)) %>%
ungroup -> data4
ggplot(data4, aes(x = items, y = n)) +
geom_bar(aes(fill = factor(answer),
color = fit_cat), position = "fill", stat = "identity") +
geom_text(aes(y = y_pos, label = n), size = 3) +
scale_color_manual(values = c("NA", "red"), guide = "none") +
scale_fill_grey()
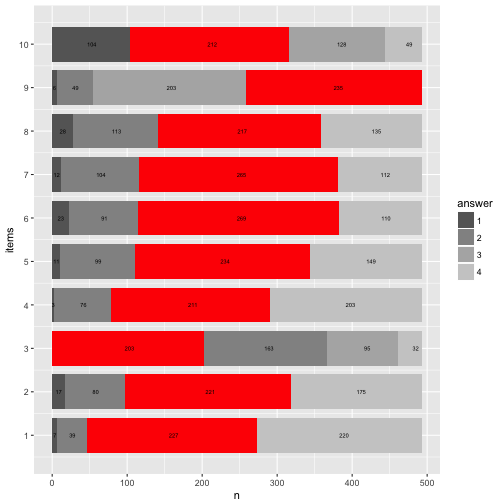
To be honest, does really look nice. Let’s try something different later.
Barplot with geom_rect
This section and code is inspired by this post.
The goal is to produce a more flexible bar plot, such as a “waterfall plot”, where y= 0 is centered at the middle bar height, we need to change the geom
. No more geom_bar
but the more versatile geom_rect
. This geom plots, surprisingly, rectangles. Hence, we need to know the start and the end value (for the y-axis, the width of the bar is just 1).
First, we need to prepare the dataset for that task.
data4 %>%
select(items, answer, y_cumsum) %>%
group_by(items) %>%
spread(key = answer, value = y_cumsum) %>%
mutate(zero = 0, end = nrow(data_items)) %>%
select(items, zero, `1`, `2`, `3`, `4`, end) %>%
ungroup %>%
mutate(items_num = 1:10) -> data5
data5 %>%
rename(start_1 = zero) %>%
mutate(end_1 = `1`) %>%
mutate(start_2 = `1`) %>%
mutate(end_2 = `2`) %>%
mutate(start_3 = `2`) %>%
mutate(end_3 = `3`) %>%
mutate(start_4 = `3`) %>%
mutate(end_4 = end) %>%
select(items_num, start_1, end_1, start_2, end_2, start_3, end_3, start_4, end_4) -> data6
head(data6) %>% kable
items_num |
start_1 |
end_1 |
start_2 |
end_2 |
start_3 |
end_3 |
start_4 |
end_4 |
1 |
0 |
7 |
7 |
46 |
46 |
273 |
273 |
493 |
2 |
0 |
175 |
175 |
396 |
396 |
476 |
476 |
493 |
3 |
0 |
203 |
203 |
366 |
366 |
461 |
461 |
493 |
4 |
0 |
203 |
203 |
414 |
414 |
490 |
490 |
493 |
5 |
0 |
11 |
11 |
110 |
110 |
344 |
344 |
493 |
6 |
0 |
110 |
110 |
379 |
379 |
470 |
470 |
493 |
OK, we have that, pooh, quite a mess.
So, now we need it in long form. Here come a not so elegant, but rather simple, solution for that.
data_cat1 <- select(data6, items_num, start_1, end_1) %>% setNames(c("items", "start", "end")) %>% mutate(answer = 1)
data_cat2 <- select(data6, items_num, start_2, end_2) %>% setNames(c("items","start", "end")) %>% mutate(answer = 2)
data_cat3 <- select(data6, items_num, start_3, end_3) %>% setNames(c("items","start", "end")) %>% mutate(answer = 3)
data_cat4 <- select(data6, items_num, start_4, end_4) %>% setNames(c("items","start", "end")) %>% mutate(answer = 4)
data7 <- bind_rows(data_cat1, data_cat2, data_cat3, data_cat4)
data7 %>% head %>% kable
items |
start |
end |
answer |
1 |
0 |
7 |
1 |
2 |
0 |
175 |
1 |
3 |
0 |
203 |
1 |
4 |
0 |
203 |
1 |
5 |
0 |
11 |
1 |
6 |
0 |
110 |
1 |
Now let’s plot the bar graph with the rectangle plot.
data7$answer <- factor(data7$answer)
ggplot(data7) +
aes() +
geom_rect(aes(x = items,
ymin = start,
ymax = end,
xmin = items - 0.4,
xmax = items + 0.4,
fill = answer)) +
scale_x_continuous(breaks = 1:10, name = "items") +
ylab("n") -> p5
p5 + geom_text(data = data3, aes(x = items_num, y = y_pos*nrow(data), label = n), size = 2) -> p6
p6
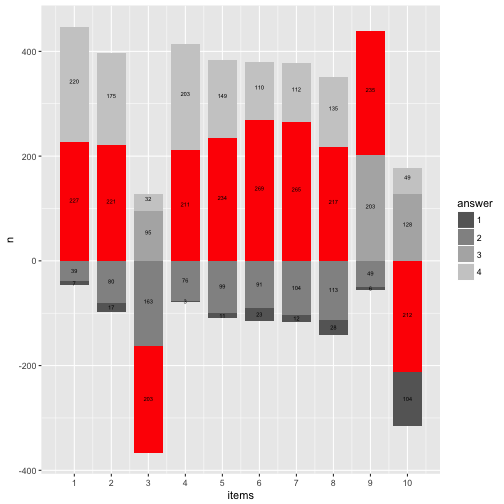
Compare that to the geom_bar
solution.
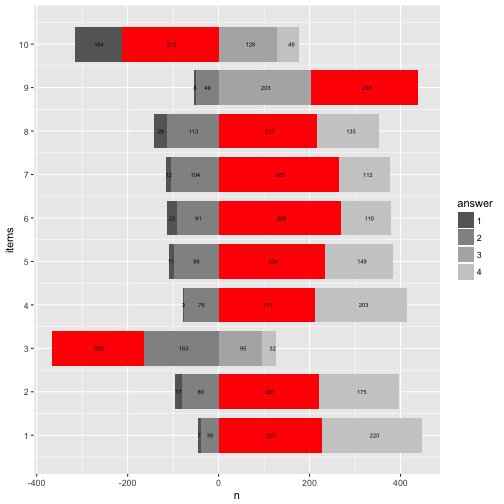
Basically identical (never mind the bar width).
Now flip the coordinates again (and compare to previous solution with geom_bar
).
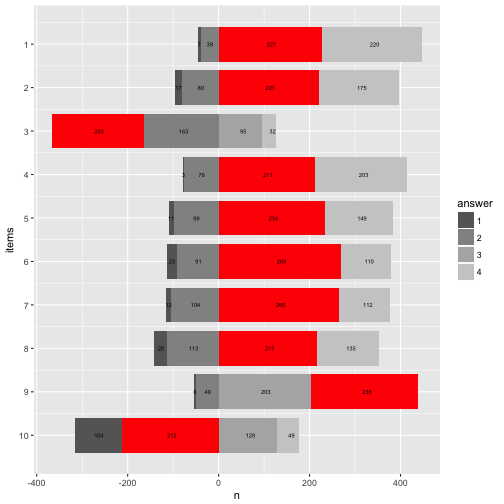
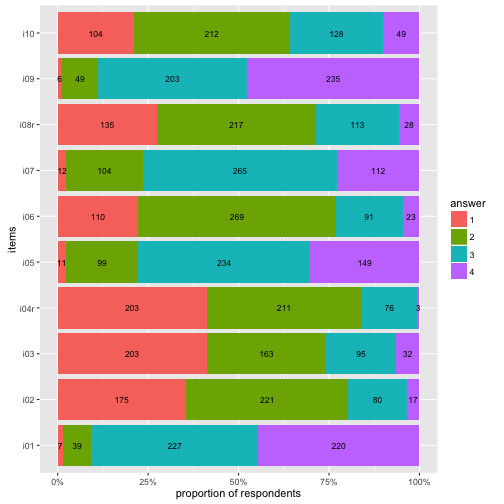
Let’s try to highlight the most frequent answer category for each item.
data7 %>%
mutate(n = end - start) %>%
group_by(items) %>%
mutate(max_n = max(n)) %>%
mutate(max_cat = factor(which(n == max_n))) %>%
mutate(fit_cat = (answer == max_cat)) %>%
ungroup -> data8
data8 %>%
filter(fit_cat == TRUE) -> data8a
data7 %>%
ggplot() +
aes() +
geom_rect(aes(x = items,
ymin = start,
ymax = end,
xmin = items - 0.4,
xmax = items + 0.4,
fill = answer)) +
scale_x_continuous(breaks = 1:10, name = "items") +
ylab("n") +
scale_fill_grey(start = .4, end = .8) -> p8
p8 + geom_rect(data = data8a, aes(x = items,
ymin = start,
ymax = end,
xmin = items - 0.4,
xmax = items + 0.4), fill = "red") +
geom_text(data = data3, aes(x = items_num, y = y_pos*nrow(data), label = n), size = 2) -> p8
p8 + coord_flip()
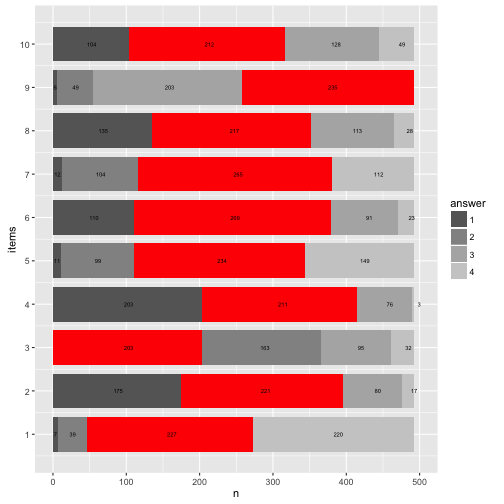
Here’s a cheat sheet on colors and palettes; and here you’ll find an overview on colors (with names and hex codes).
Mrs. Brewer site is a great place to come up with your own palette and learn more about colors.
Rainbow diagram
Let’s try to “move” the bars to our wishes. Precisely, it would be nice if the bars were aligned at the “Rubicon” between “do not agree” and “do agree”. Then we could see better how many persons agree and not.
More practically, if we knew that 104+212 = 316 persons basically disagree, then 316 could be our “zero line” (item 10). Repeat that for each item.
data8 %>%
group_by(items) %>%
mutate(n_disagree = sum(n[c(1,2)]),
n_agree = sum(n[c(3,4)]),
start_adj = start - n_disagree,
end_adj = end - n_disagree
) %>%
ungroup -> data9
data9 %>%
ggplot() +
aes() +
geom_rect(aes(x = items,
ymin = start_adj,
ymax = end_adj,
xmin = items - 0.4,
xmax = items + 0.4,
fill = answer)) +
scale_x_continuous(breaks = 1:10, name = "items") +
ylab("n") +
scale_fill_grey(start = .4, end = .8) -> p9
p9
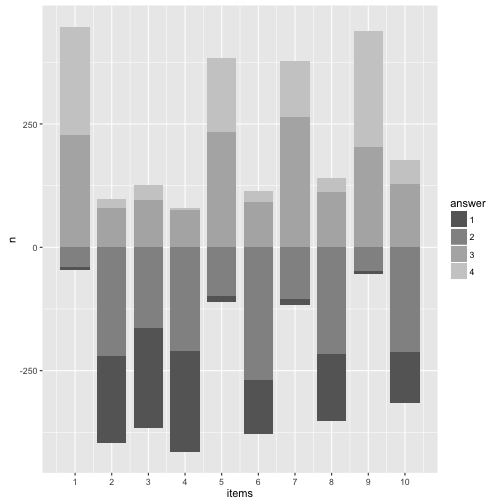
Looks quite cool.
Now let’s add the numbers to that plot.
data4 %>%
mutate(items_num = parse_number(items)) %>%
dplyr::select(-items) %>%
rename(items = items_num) %>%
select(items, everything()) %>%
arrange(answer, items) %>%
ungroup -> data10
data9 %>%
mutate(y_pos_adj = data10$y_pos * nrow(data) - n_disagree) -> data11
p9 +
geom_text(data = data11, aes(x = items, y = y_pos_adj, label = n), size = 2) -> p11
p11
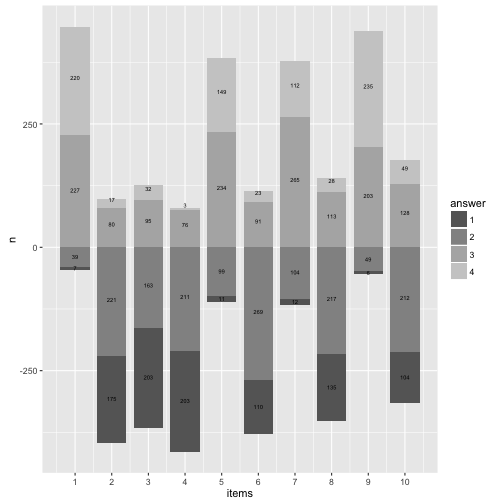
And now let’s highlight the most frequent answer category.
library(ggrepel)
data11 %>%
ggplot() +
aes() +
geom_rect(aes(x = items,
ymin = start_adj,
ymax = end_adj,
xmin = items - 0.4,
xmax = items + 0.4,
fill = answer)) +
scale_fill_grey(start = .4, end = .8) +
geom_rect(data = filter(data11, fit_cat == TRUE),
aes(x = items,
ymin = start_adj,
ymax = end_adj,
xmin = items - 0.4,
xmax = items + 0.4),
fill = "red") +
geom_text(aes(x = items, y = y_pos_adj, label = n), size = 2) +
scale_x_continuous(breaks = 1:10) +
ylab("n") -> p12
p12
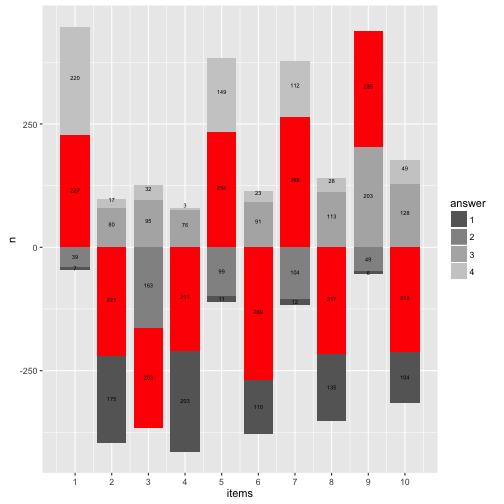
OK, that’s about it for today. May turn the waterfall by 90°.
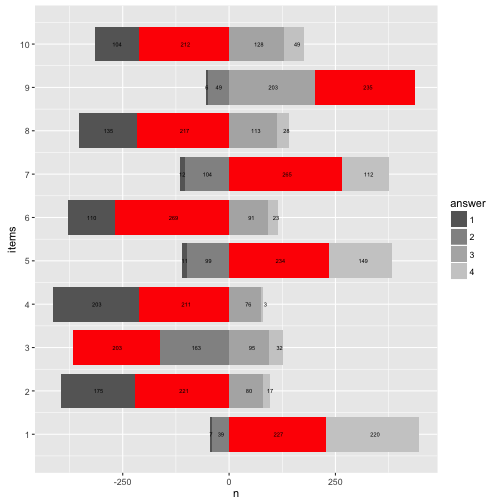
And change the direction of the now numeric items-axis.
p12 + coord_flip() + scale_x_reverse(breaks = 1:10)
## Scale for 'x' is already present. Adding another scale for 'x', which
## will replace the existing scale.
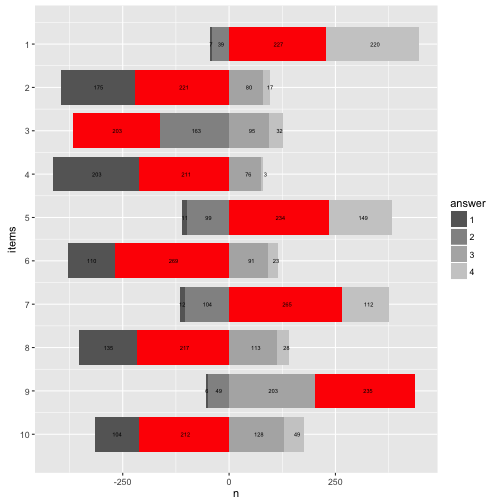
Happy plotting!